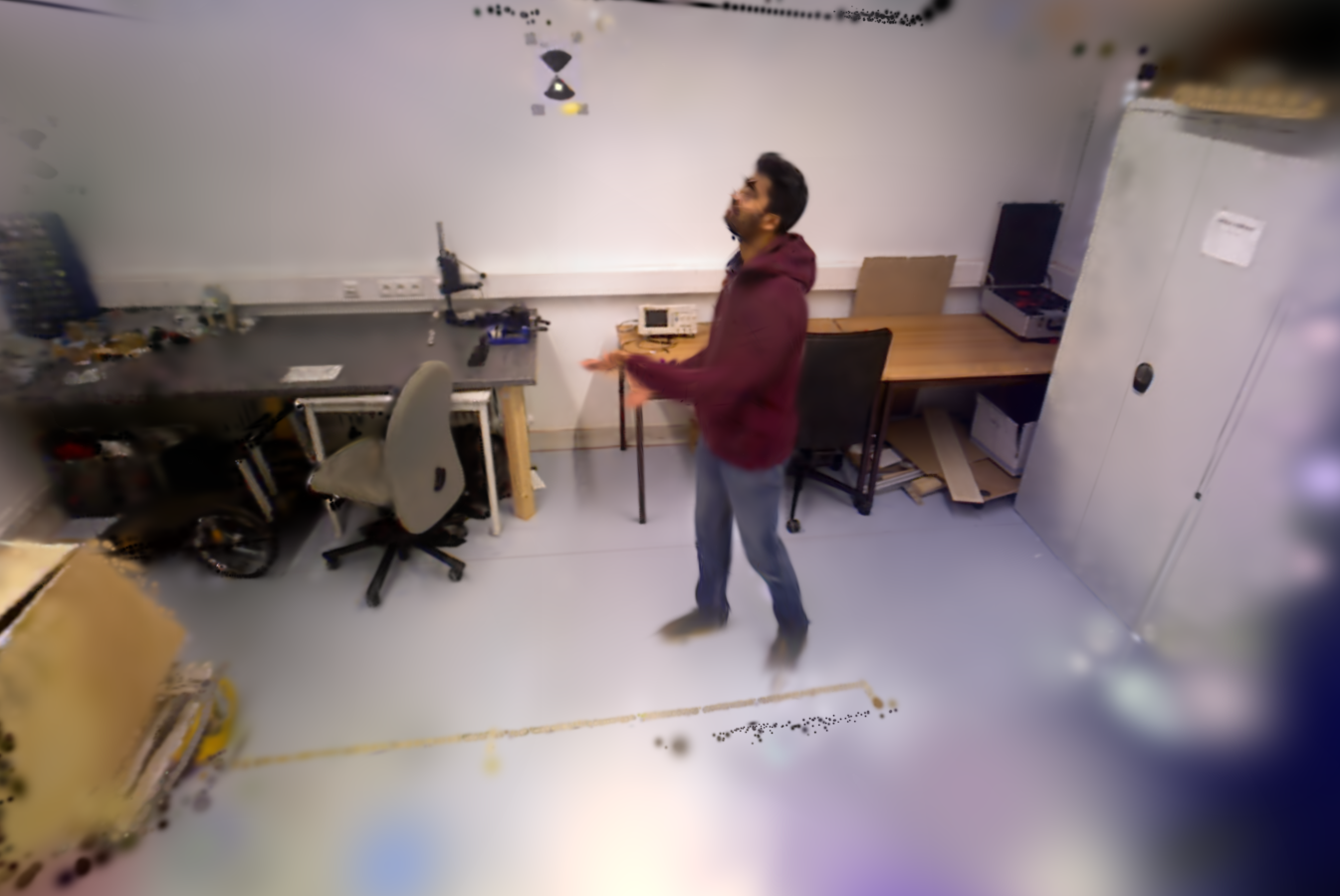
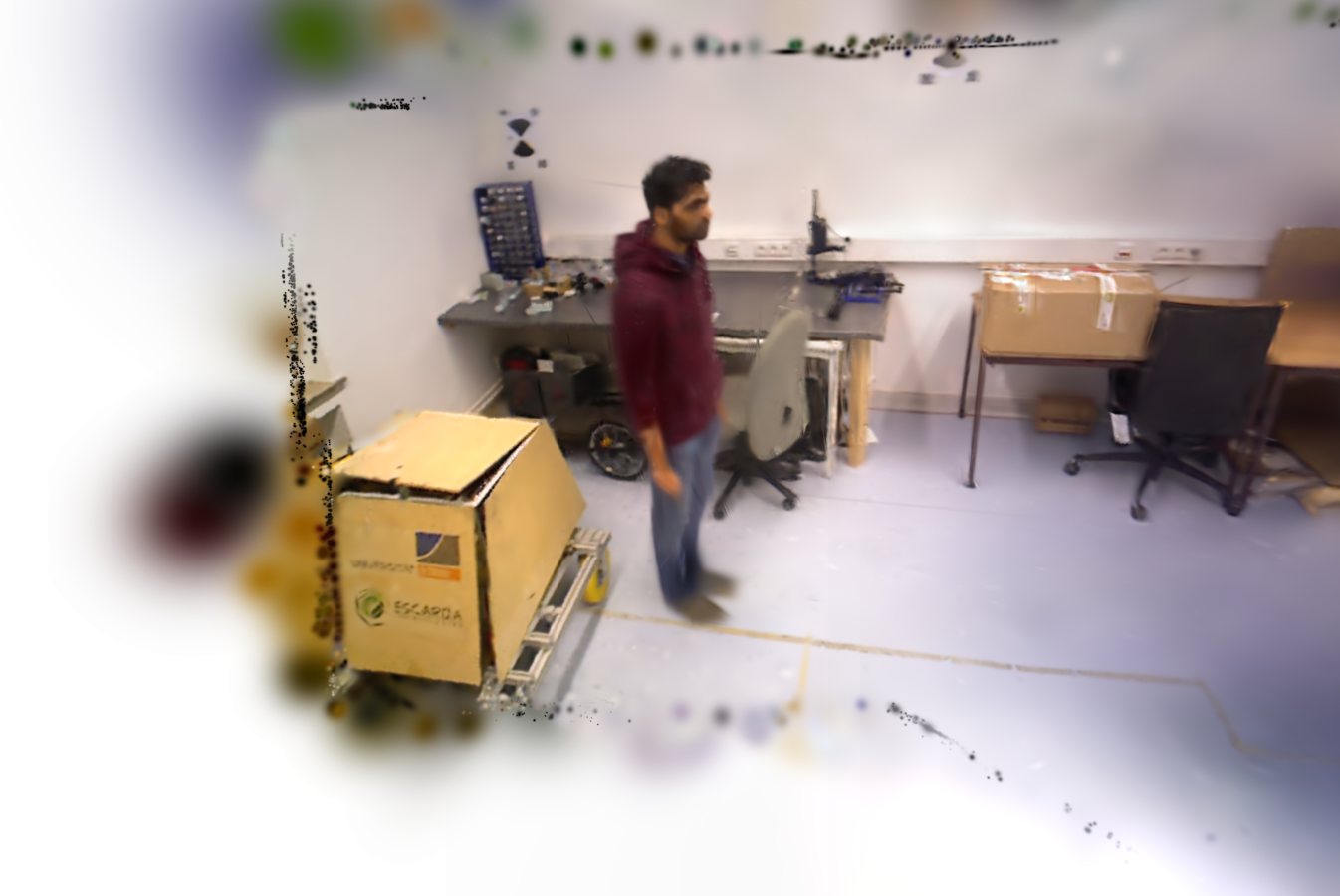
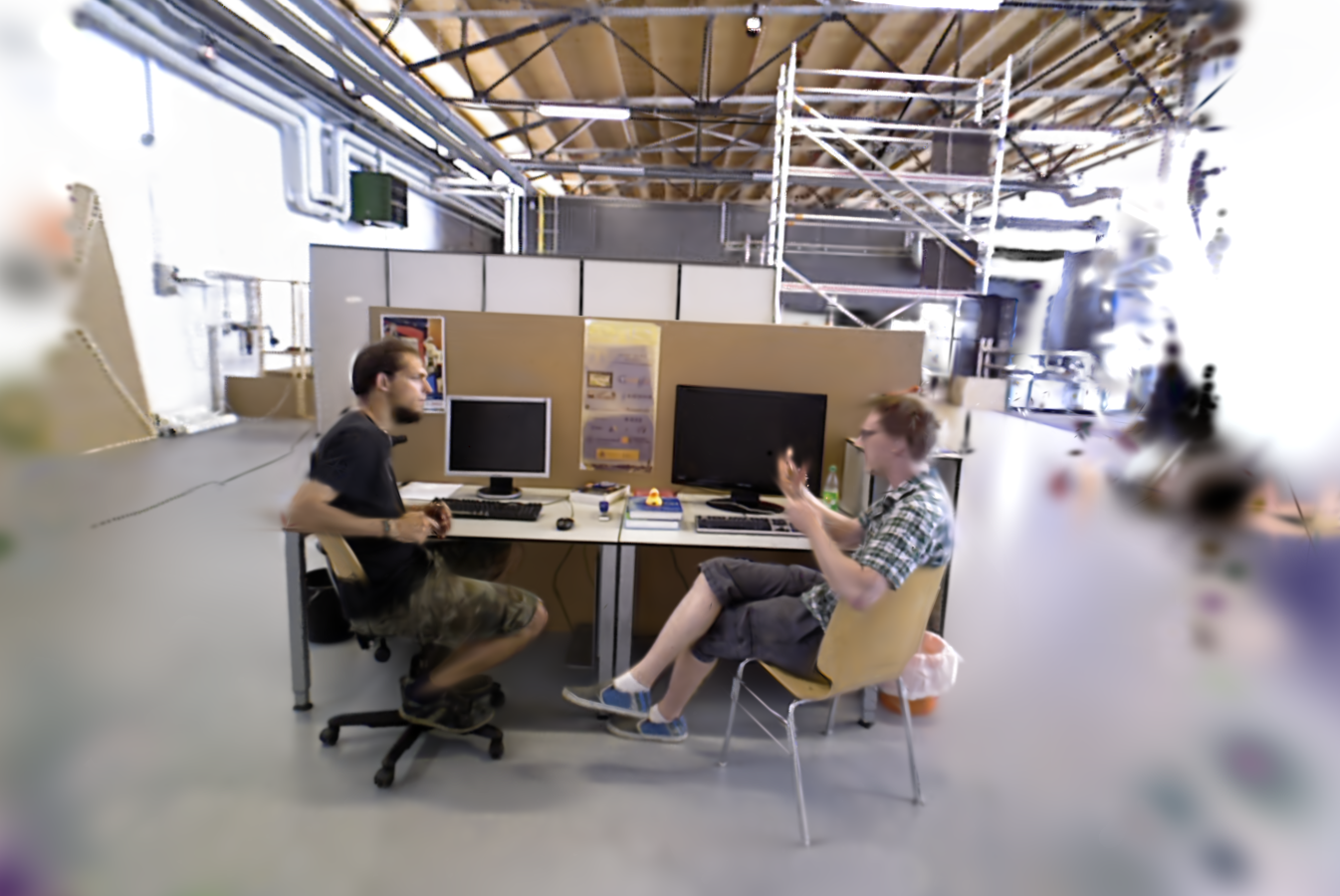
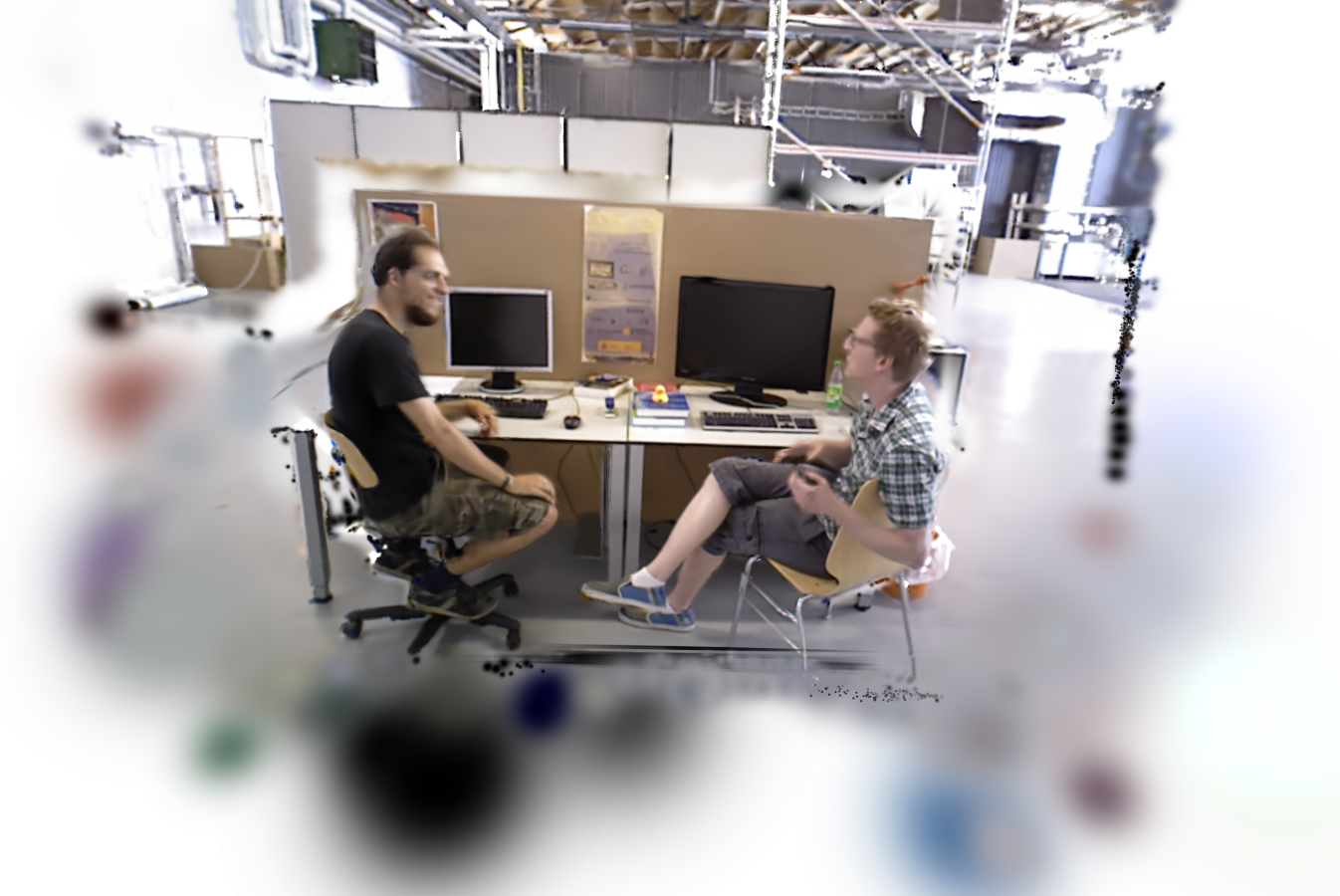
Instead of removing dynamic objects as distractors and reconstructing only static environments, this paper proposes an efficient architecture that incrementally tracks camera poses and establishes the 4D Gaussian radiance fields in unknown scenarios by using a sequence of RGB-D images. First, by generating motion masks, we obtain static and dynamic priors for each pixel. To eliminate the influence of static scenes and improve the efficiency on learning the motion of dynamic objects, we classify the Gaussian primitives into static and dynamic Gaussian sets, while the sparse control points along with an MLP is utilized to model the transformation fields of the dynamic Gaussians. To more accurately learn the motion of dynamic Gaussians, a novel 2D optical flow map reconstruction algorithm is designed to render optical flows of dynamic objects between neighbor images, which are further used to supervise the 4D Gaussian radiance fields along with traditional photometric and geometric constraints.
@misc{li20254dgaussiansplattingslam,
title={4D Gaussian Splatting SLAM},
author={Yanyan Li and Youxu Fang and Zunjie Zhu and Kunyi Li and Yong Ding and Federico Tombari},
year={2025},
eprint={2503.16710},
archivePrefix={arXiv},
primaryClass={cs.CV},
url={https://arxiv.org/abs/2503.16710},
}